AI-Vision Evolution:
From Neural Networks to Seeing Machines
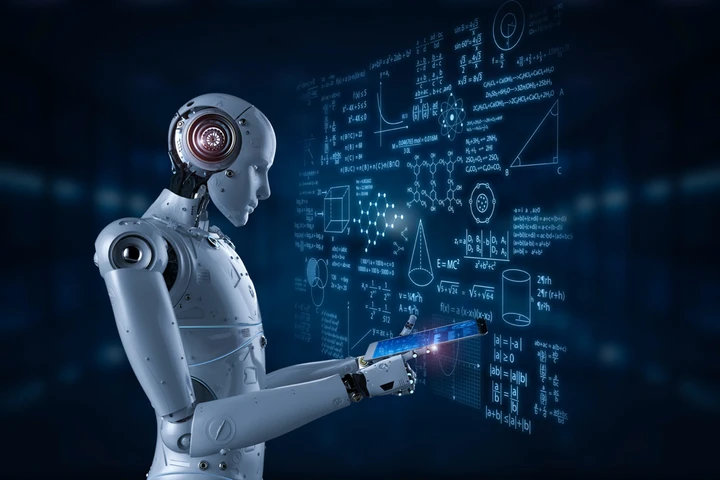
The Dawn of Neural Networks
The journey of AI-Vision began in the mid-20th century with the conceptualization of neural networks. Inspired by the workings of the human brain, early computer scientists envisioned creating machines capable of learning and recognizing patterns. The 1950s and 1960s saw the development of the first neural network models. These were rudimentary by today’s standards, but they laid the groundwork for what was to come. The Perceptron, developed by Frank Rosenblatt in 1957, was one of the first. It was designed to recognize simple patterns, a precursor to the complex image recognition tasks performed by AI today.
The AI Winter and Resurgence
Despite early enthusiasm, progress in AI and neural networks faced significant hurdles, leading to periods known as “AI winters.” The limitations of computing power and the complexity of neural network models led to a temporary decline in interest and funding. However, the 1980s and 1990s brought a resurgence, thanks to increased computational capabilities and the development of new algorithms. The invention of backpropagation, for example, allowed neural networks to adjust and improve through learning, paving the way for more advanced AI applications.
The Advent of Machine Vision
As AI research progressed, a new field emerged: machine vision. This field focused on giving computers the ability to see and interpret the world. Early machine vision systems were employed in industrial settings, such as for inspecting products on assembly lines. These systems, while basic, demonstrated the potential of integrating visual processing with AI.
Breakthroughs in Deep Learning
The 21st century ushered in an era of unprecedented growth in AI, particularly with the advent of deep learning. Deep learning networks, characterized by their deep layers of neural processing, enabled machines to recognize and interpret complex visual data with remarkable accuracy. This period saw the development of convolutional neural networks (CNNs), which are specifically designed for processing visual data. They can identify faces, interpret scenes, and even generate realistic images.
The Future of AI-Vision
Today, AI-Vision stands at the forefront of technological advancement. Autonomous vehicles utilize this technology for road navigation, drones for aerial reconnaissance and surveying, and the healthcare industry employs it in disease diagnosis through medical imagery. The potential of AI-Vision is boundless, with ongoing research continually expanding the limits of what AI can perceive and comprehend.
However, despite cameras’ ability to see and sometimes even surpass human capabilities in interpreting visual information, a significant gap remains between AI-Vision technology and ready-to-use business solutions that align with existing business processes. Merely recognizing objects in a video stream is not enough. The real challenge lies in making informed decisions and taking actions, ideally autonomously. This is where Anymatica steps in. As a leading AI-Vision Integration platform, Anymatica bridges the gap between AI-Vision technology and integrated business solutions. It achieves this swiftly and seamlessly, utilizing a no-code engine to craft adaptive business scenarios for any case, from building supervision to developing autonomous agents.